文本和单图如何生成无缝的360度全景图像?

我们非常重视原创文章,为尊重知识产权并避免潜在的版权问题,我们在此提供文章的摘要供您初步了解。如果您想要查阅更为详尽的内容,访问作者的公众号页面获取完整文章。

Summary
Unlike traditional 2D images, 360-degree panorama images capture the entire 360°×180° field of view, posing a significant challenge in ensuring the right and left edges are seamlessly connected. Current diffusion processes are inadequate for creating such seamless panoramas. To address this, the authors introduce a method that uses a circular blending strategy during both denoising and VAE decoding to maintain geometric continuity. This approach has led to the development of two models for text-to-360-panorama and single-image-to-360-panorama tasks. The code is openly available at GitHub.
Related Work
Recent research like MVDiffusion, StitchDiffusion, and PanoDiff has demonstrated the potential for diffusion-based 360-degree panoramic image generation, yet these methods have their shortcomings. MVDiffusion relies on eight perspective views and results in wide-angle distant images with artifacts. StitchDiffusion maintains continuity through global trimming but still leaves visible seams upon magnification. PanoDiff, the most closely related work, introduced a circular padding scheme. The authors' method differs by employing an adaptive weighting strategy for geometric continuity, simplifying the training process by avoiding the need for a Rotating Schedule, and allowing direct application of their technique to the ControlNet-Tile model for high-resolution results.
Method
The authors propose a circular blending strategy applied during inference to create seamless 360-degree panoramas. This involves adaptively blending the right side of the latent features with the left side during each denoising step. Similarly, this strategy is integrated into the VAE decoder's tiled_decode function, with a greater emphasis on maintaining geometric continuity during VAE decoding.
Text-to-360 Panorama
For text-to-360-panorama tasks, a multi-stage framework is suggested for generating high-resolution 360-degree panoramas. Starting with a low-resolution image generated by a base model fine-tuned using the DreamBooth method on the SUN360 dataset, various super-resolution strategies are employed, including diffusion-based and GAN-based techniques like ControlNet-Tile and RealESRGAN. Further fine-tuning of the ControlNet-Tile model on the SUN360 dataset enhances the results.
Single Image to 360 Panorama
The framework for single-image-to-360-panorama tasks mirrors the text-to-360 approach but substitutes the base model with the controlnet-outpainting model. The ControlNet-Outpainting model generates low-resolution 360-degree panoramas from a single perspective view of a 2D image. Training pairs of perspective and panorama images are created by transforming panoramic images into cubic maps. Unfortunately, the training model for this task cannot be released, but it should be easily reproducible.
Results and Limitations
Several test results for different stages of the text-to-360-panorama task are presented, demonstrating the effectiveness of the approach. However, there are limitations as the base model is trained with DreamBooth, prohibiting changes to a CIVITAI model for stylization. While initial 360 images can be generated with the method, styles cannot be altered by adding descriptors to the prompts but require different base models and ControlNets.
References
The paper referenced is "Diffusion360: Seamless 360 Degree Panoramic Image Generation based on Diffusion Models". Available at arXiv. The authors invite exchanges with AI artists in the field.
想要了解更多内容?

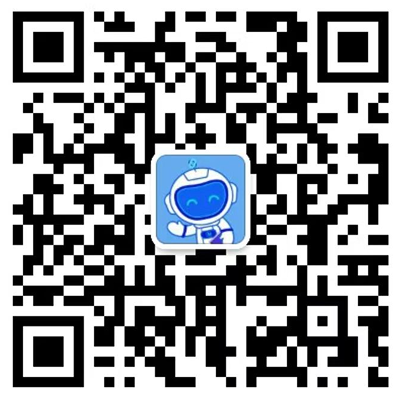
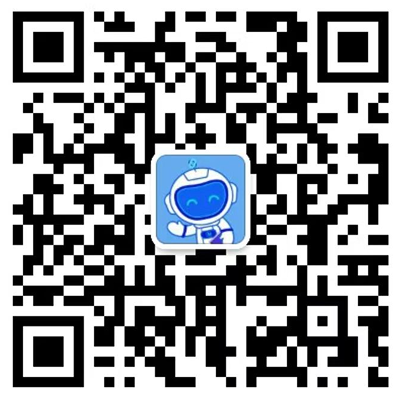
白皮书上线