一文详解视频扩散模型的最新进展

我们非常重视原创文章,为尊重知识产权并避免潜在的版权问题,我们在此提供文章的摘要供您初步了解。如果您想要查阅更为详尽的内容,访问作者的公众号页面获取完整文章。


Video Diffusion Models: A Survey
Introduction
This survey thoroughly examines the latest developments in the era of AI-Generated Content (AIGC), with a focus on video diffusion models. It presents a comprehensive review of over 100 works that address tasks in video generation, editing, and understanding, offering a structured categorization based on their technical perspectives and research objectives. Furthermore, the survey outlines the fundamental concepts of diffusion processes, popular benchmark datasets, and commonly used evaluation metrics. Experimental setups are described in detail, with fair comparative analyses conducted across various benchmark datasets. Finally, the survey suggests several directions for future research in video diffusion models.
Challenges and Future Trends
- Collection of Large-Scale Video Text Datasets: There is an urgent need for improved datasets in terms of size, annotation accuracy, and video quality to match the significant achievements in text-to-image synthesis.
- Efficient Training and Inference: The considerable training costs of T2V models pose a significant challenge, and research into more efficient model training and reduced inference times is a valuable direction for future research.
- Benchmarking and Evaluation Methods: Current metrics like FVD and IS emphasize the difference between the distribution of generated videos and real videos, highlighting the need for more comprehensive evaluation benchmarks and metrics that accurately reflect the quality of video generation.
- Insufficient Model Capacity: Many current methods still fail in certain scenarios due to the inherent limitations of existing image generation models, and further research and enhancements are key to overcoming these limitations.
Conclusion
The survey offers a deep dive into the state-of-the-art of video diffusion models in the AIGC era, marking the first attempt of its kind. It provides a foundational understanding of diffusion processes, benchmarks, and evaluation techniques, alongside a detailed review and categorization of works focused on video generation, editing, and understanding tasks. The experimental section delivers an in-depth description of experimental setups and fair comparative analyses across benchmarks. Lastly, it proposes several recommendations for future research directions in video diffusion models.
想要了解更多内容?







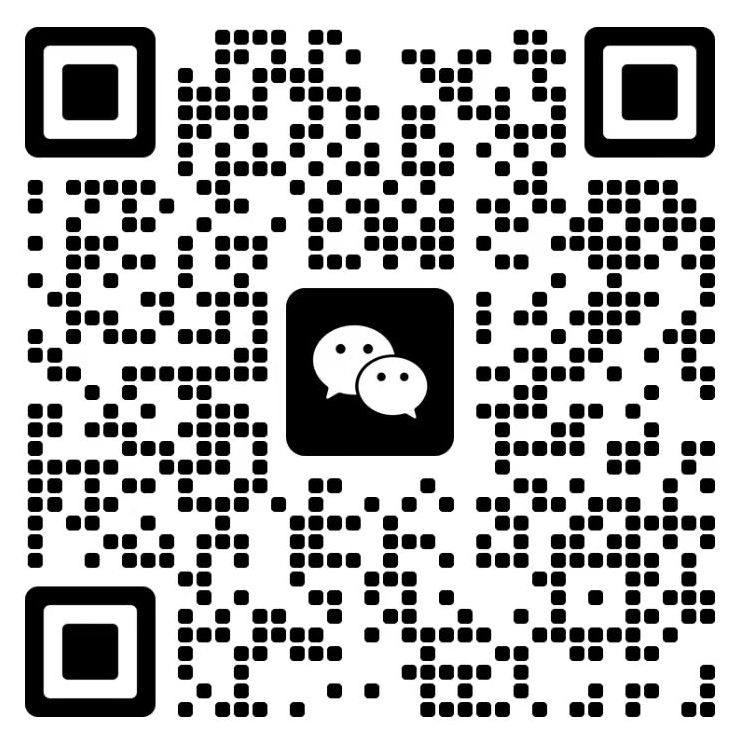
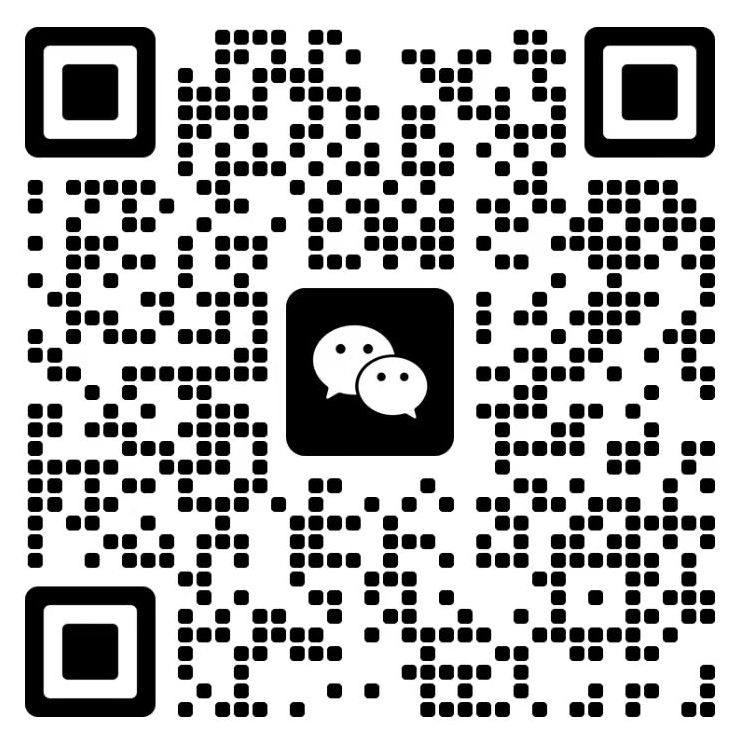