海报生成如此简单!OPPO联合港中文发布基于LLM的端到端方案GlyphDraw2

我们非常重视原创文章,为尊重知识产权并避免潜在的版权问题,我们在此提供文章的摘要供您初步了解。如果您想要查阅更为详尽的内容,访问作者的公众号页面获取完整文章。

Summary
Introduction: This article introduces an end-to-end text rendering framework for generating complex glyph posters, which plays a vital role in marketing and advertising by enhancing visual communication and brand recognition. Despite improvements in text rendering accuracy, the field of end-to-end poster generation remains underexplored. The proposed framework employs a triple cross-attention mechanism rooted in alignment learning, a high-resolution dataset exceeding 1024 pixels, and leverages the SDXL architecture to create posters with detailed contextual backgrounds.
Contributions: The paper's main contributions include an end-to-end poster generation solution that fine-tunes large language models (LLMs) for layout planning, a glyph generation framework based on alignment learning and triple cross-attention that accurately places text while maintaining a visually rich background, and the introduction of a high-resolution dataset with Chinese and English glyph image pairs.
Methodology: The framework consists of four parts: Fusion Text Encoder (FTE) for integrating modal features, Triples of Cross-Attention (TCA) with two different attention layers in the SD decoder to enhance glyph rendering accuracy and layout, Auxiliary Alignment Loss (AAL) learning to enhance overall layout and enrich poster background information, and fine-tuned LLM strategy for inferring user descriptions to generate glyph and condition framework coordinates.
Experimental Details: The model is trained in two main components: a controllable text-to-image poster model based on SDXL with multi-language understanding capability and a layout generation model based on LLMs fine-tuned specifically for poster data. The model has been trained on 64 A100 GPUs with a batch size of 2 for 100,000 steps, and the LLM layout generation model has been trained for 30,000 steps with a batch size of 10.
Evaluation: The evaluation set is divided into two parts: AnyText-Benchmark and two subsets including Complex-Benchmark and Poster-Benchmark. The evaluation leverages various metrics, such as Position Word Accuracy (PWAcc), Normalized Edit Distance (NED), accuracy, ClipScore, and HPSv2, to assess text rendering quality and image preferences.
Results: The proposed model demonstrates superior performance in generating posters with complex and context-rich backgrounds, outperforming existing methods on various evaluation sets and showing potential as a foundation for enhanced end-to-end poster generation capabilities.
Conclusion and Limitations: While the method can generate posters with free resolution end-to-end, there are still issues such as low prediction accuracy for LLM-predicted glyph bboxes in complex scenarios and the balance between background richness and text rendering accuracy. Future work might explore solutions within the text encoder to address these problems.
想要了解更多内容?

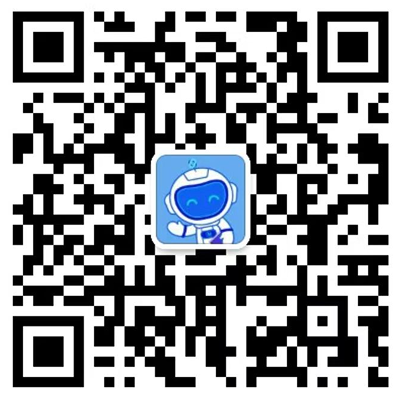
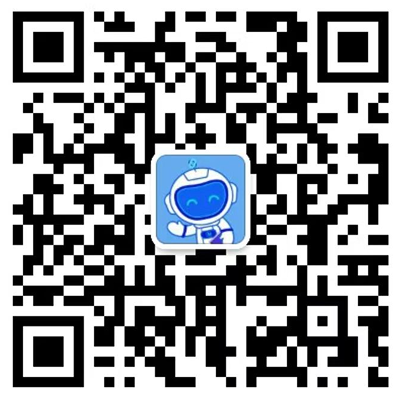
白皮书上线