为Stable Diffusion模型瘦身并达到SOTA!LAPTOP-Diff:剪枝蒸馏新高度(OPPO)

我们非常重视原创文章,为尊重知识产权并避免潜在的版权问题,我们在此提供文章的摘要供您初步了解。如果您想要查阅更为详尽的内容,访问作者的公众号页面获取完整文章。

Summary
Introduction
Stable Diffusion Model (SDM) plays a critical role in the AI-generated content (AIGC) community but faces deployment challenges on personal and mobile devices due to high memory consumption and latency. Various methods have been proposed to reduce the inference budget of SDMs, including architecture design and structural pruning. However, these methods often lack efficiency, scalability, and generalizability. To address these issues, this paper introduces a layer pruning and normalized distillation method for compressing diffusion models, termed LAPTOP-Diff.
Related Work
This article discusses the existing diffusion models and highlights the impact of Stable Diffusion in generating high-quality images. The paper also reviews efficient architecture design methods and layer pruning approaches, revealing the shortcomings of manual layer removal and the potential of layer pruning for better scalability and performance.
Methodology
LAPTOP-Diff proposes an automatic layer pruning method to compress SDM's U-Net by introducing a one-time pruning criterion based on a combination optimization perspective, which outperforms other layer pruning and manual removal methods. It also addresses the imbalance issue in feature distillation during retraining by employing normalized feature distillation, leading to significant performance improvements even with a 50% pruning ratio.
Experiments
LAPTOP-Diff's effectiveness is demonstrated through extensive experiments and comparisons with state-of-the-art compression methods for SDMs, achieving minimal performance drop with significant pruning ratios. The approach is further validated through ablation studies and analysis, confirming that LAPTOP-Diff provides a scalable, automated, and high-performance solution for compressing diffusion models.
Conclusion
The paper concludes that LAPTOP-Diff offers an effective solution for compressing diffusion models with its layer pruning and normalized distillation approach, achieving state-of-the-art performance compression for SDMs and paving the way for low-cost and device-end applications of diffusion models in the AIGC era.
想要了解更多内容?

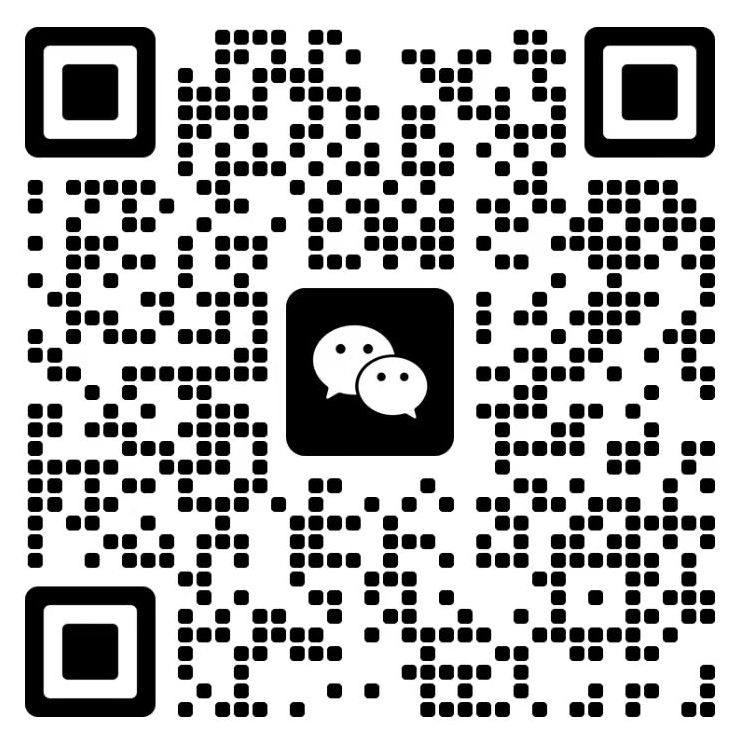
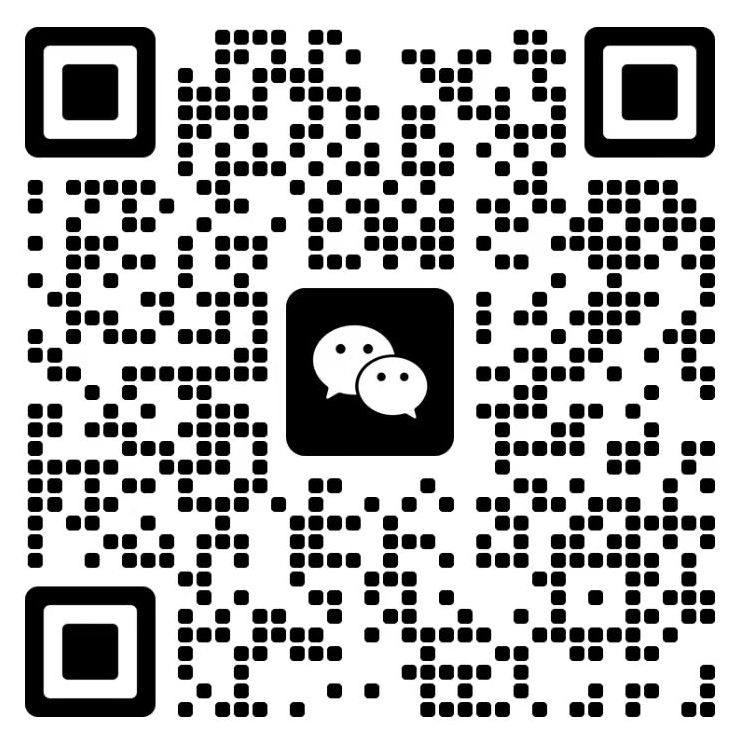