如何"正确"使用Stable Diffusion?文本到图像扩散模型中记忆化实用分析(浙大)

我们非常重视原创文章,为尊重知识产权并避免潜在的版权问题,我们在此提供文章的摘要供您初步了解。如果您想要查阅更为详尽的内容,访问作者的公众号页面获取完整文章。

Summary
The paper presents a practical analysis method for understanding memorization in text-to-image diffusion models. It introduces a formal definition of image memorization and identifies three necessary conditions for memorization: similarity, existence, and probabilistic nature. A correlation between model prediction error and image duplication is revealed, which leads to the proposal of inversion techniques to assess and measure the extent of memorization.
Introduction
Diffusion probabilistic models have shown impressive capabilities in various applications. Despite their popularity, concerns about copyright and privacy violations arise due to the training datasets containing potentially sensitive content. This work focuses on the memorization issue within text-to-image diffusion models, which can lead to the rigid generation of identical data from the training set, a failure against the expected novelty and diversity of a probabilistic generation model.
Background
Diffusion models are latent variable models that use a denoising process to generate images. The training for state-of-the-art models involves large-scale datasets that often contain copyrighted or private content. The paper examines memorization in diffusion models since they can potentially replicate training data, which poses legal and ethical issues.
Definition of Memorization
The paper formally defines memorization in diffusion models, considering similarity to target images, existence of a prompt that triggers duplication, and the probabilistic nature of frequent replication during sampling. It uses model prediction error as a metric to identify image duplication and proposes a prompt inversion algorithm for analyzing the existence condition.
Identification of Image Duplication
A preliminary investigation into image duplication within diffusion models is presented, using prediction error as a measure for identifying image copies. The paper outlines a methodology for inverting noise-prompt pairs to verify if they replicate a target image.
Measuring Memorization
The paper discusses methods for measuring memorization by comparing the distribution of model errors when sampling with and without specific prompts. It utilizes an unconditional diffusion model trained on large-scale datasets as a reference for a safe model, against which memorization can be measured.
Related Work
Previous works on memorization in image generation models have focused on unconditional generation types, such as GANs and VAEs. The paper extends this to conditioned diffusion models and leverages inversion techniques originally used for image editing to analyze memorization in training data.
Discussion and Conclusion
The paper provides a practical tool for developers to analyze the safety of a set of sensitive training images against memorization. The contributions include a practical analysis of memorization, a formal definition with measurable conditions, and detailed experiments on the Stable Diffusion model to validate the proposed analysis methods. Limitations and potential directions for future work are also discussed, including developing more robust prompt inversion algorithms and extending the analysis to other types of conditional models.
Reference: Zhe Ma et al., "Could It Be Generated? Towards Practical Analysis of Memorization in Text-To-Image Diffusion Models"
想要了解更多内容?






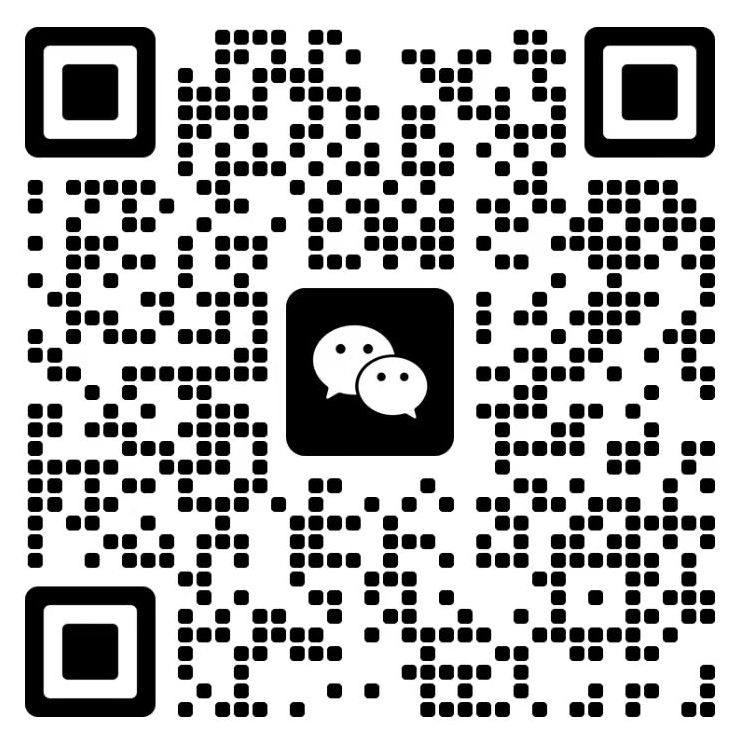
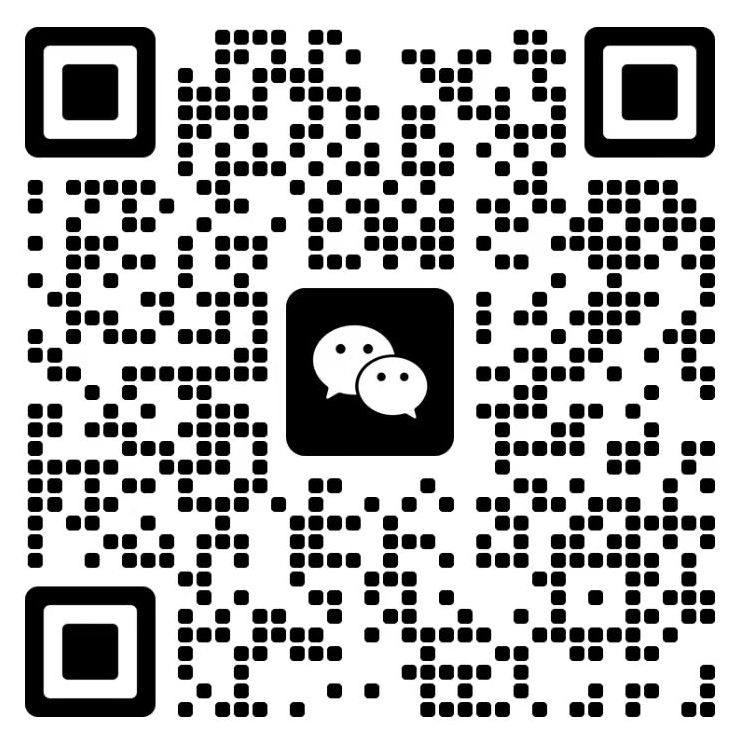
白皮书上线