IJCAI2024 | 拒绝偏见!Graph Transformers 公平性最新研究

我们非常重视原创文章,为尊重知识产权并避免潜在的版权问题,我们在此提供文章的摘要供您初步了解。如果您想要查阅更为详尽的内容,访问作者的公众号页面获取完整文章。

Summary of "FairGT: Fairness-aware Graph Transformers"
Authors: Renqiang Luo et al.
Source: AI Generation Future
Paper link: https://arxiv.org/pdf/2404.17169
Introduction
Graph Transformers (GTs) have been successful in addressing challenges faced by Graph Neural Networks (GNNs); however, they tend to overlook fairness considerations, leading to biased outcomes towards sensitive subgroups. Traditional fairness-aware graph learning methods do not directly apply to GTs due to their unique architecture that encodes graph information without relying on message passing mechanisms. To tackle this challenge, this paper introduces FairGT, a fairness-aware Graph Transformer designed to mitigate inherent fairness issues in GTs through a structured feature selection strategy and a multi-hop node feature integration method.
Related Work
While Graph Transformers have shown effectiveness in global receptive fields on graphs, current approaches generally lack explicit consideration of algorithmic fairness. On the other hand, fairness-aware GNNs have received considerable attention, focusing on pre-processing and in-processing methods. However, these solutions are not directly applicable to the unique challenges posed by GTs.
FairGT Design
FairGT enhances fairness by ensuring the independence of sensitive features during training. It introduces a novel structured topology encoding through feature vector selection from the adjacency matrix and a fairness-aware node feature encoding method. Theoretical analysis is provided to demonstrate the effectiveness of FairGT's approach in maintaining the independence of sensitive features.
Experiments
Empirical evaluations on five real-world datasets show that FairGT outperforms existing GTs, GNNs, and state-of-the-art fairness-aware graph learning methods in terms of fairness metrics. Ablation studies further highlight the significance of feature vector selection and multi-hop sensitive information integration in improving fairness outcomes.
Conclusion
The paper presents FairGT, an innovative approach towards enhancing fairness in GTs, built upon two fairness-aware graph information encodings. Despite some limitations, such as sensitivity to parameter settings, FairGT sets a foundation for the continuous development of fairness-aware GTs and their broader application in real-world scenarios.
想要了解更多内容?

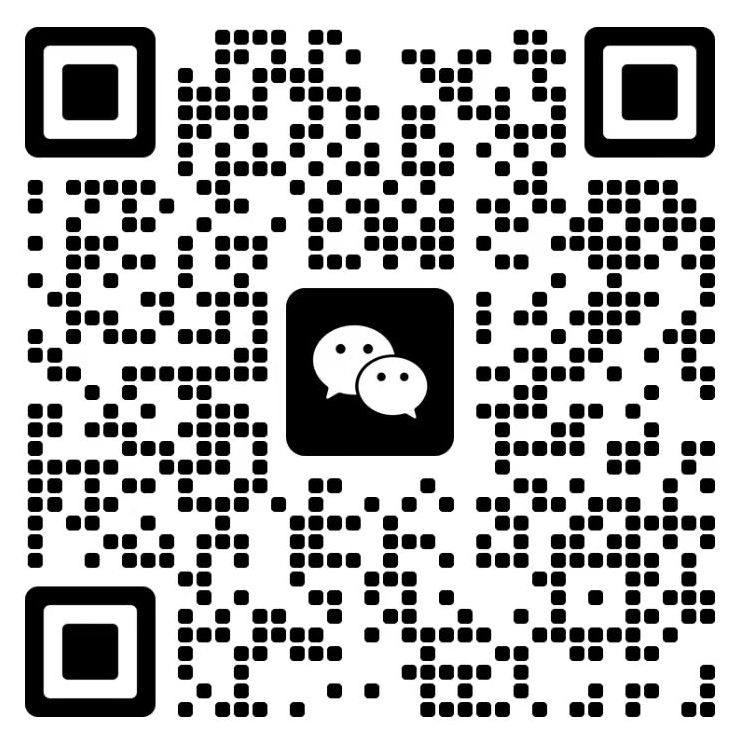
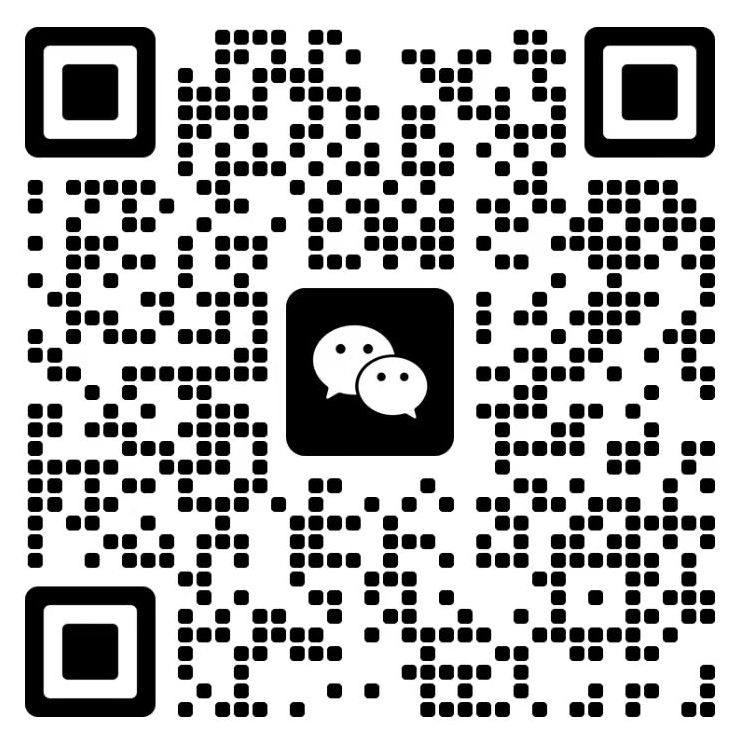
白皮书上线