迈向统一扩散框架!Adobe提出RGB↔X:双重利好下游编辑任务 | SIGGRAPH'24

我们非常重视原创文章,为尊重知识产权并避免潜在的版权问题,我们在此提供文章的摘要供您初步了解。如果您想要查阅更为详尽的内容,访问作者的公众号页面获取完整文章。

Article Summary
Introduction
Recent research indicates that the domains of forward rendering, per-pixel inverse rendering, and generative image synthesis, while seemingly disparate, are interconnected within the graphics and vision fields. The study explores the connection between diffusion models, rendering, and intrinsic channel estimation, focusing on material/light estimation and image synthesis based on material/light, all within a unified diffusion framework. This approach has led to improved estimation of intrinsic channels—albedo, roughness, metallicity (RGB→X problem)—and the synthesis of realistic images from intrinsic channels (X→RGB problem) within the diffusion framework.
Intrinsic Channels and Data Sets
The models use intrinsic channels such as albedo, normal vectors, roughness, metallicity, and diffuse irradiance, with the channels estimated in full resolution. Multiple datasets were used and extended with synthetic and real data to train the models, leading to improved scene property extraction and highly realistic image generation of indoor scenes.
RGB→X Model
The RGB→X model estimates intrinsic channels from input RGB images, using a pre-trained text-to-image latent space diffusion model, Stable Diffusion2.1. The model operates in latent space and is trained using a loss function with v-prediction. It also uses text prompts as "switches" to control which intrinsic channel is generated, improving performance significantly.
X→RGB Model
The X→RGB model performs realistic RGB image synthesis from intrinsic channels. It fine-tunes the diffusion model starting from Stable Diffusion2.1 and addresses various factors such as heterogeneous data handling and low-resolution lighting for illumination control. The model can generate images with partial information and use text prompts for extra control.
Results
The results demonstrate that the proposed models can estimate intrinsic channels and synthesize images with high fidelity, closely matching path-tracing references. The models can also generate images by specifying only part of the appearance properties and allowing the model to freely invent reasonable versions of the rest.
Applications
The combined use of RGB→X and X→RGB models enables applications like material editing and object insertion with realistic outcomes. The framework is a step toward a unified diffusion framework that can perform image decomposition and rendering, potentially benefiting a wide range of downstream editing tasks.
Conclusion
The paper presents a unified diffusion-based framework for realistic image analysis (estimation of intrinsic channels describing geometry, materials, and illumination) and synthesis (realistic rendering given intrinsic channels), demonstrated within the domain of realistic indoor scene images.
想要了解更多内容?

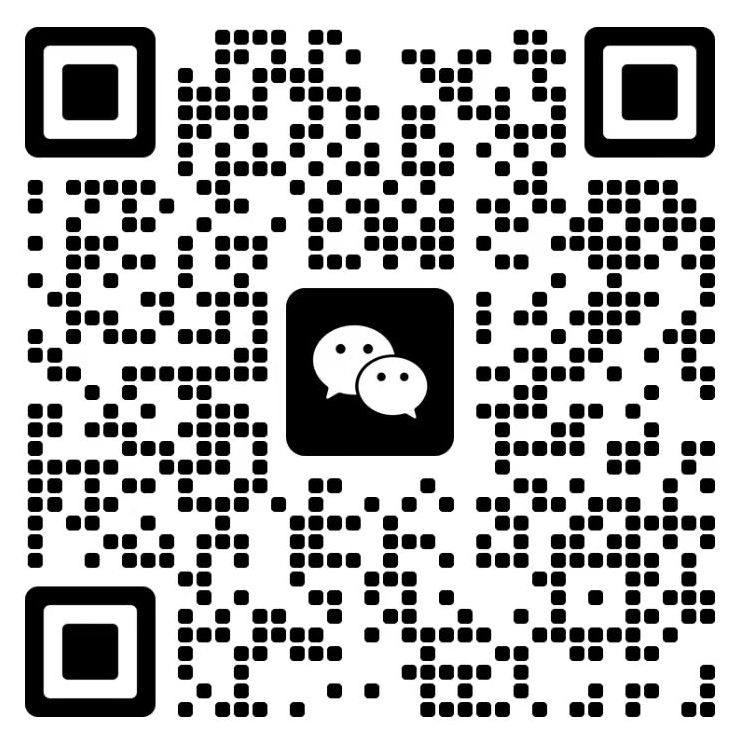
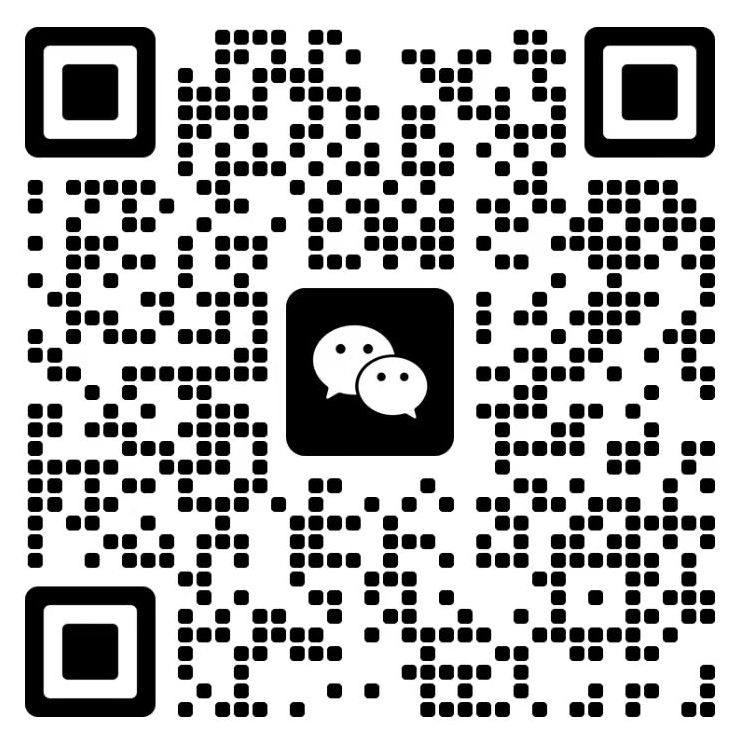