深入探讨基于大语言模型的数据标注

我们非常重视原创文章,为尊重知识产权并避免潜在的版权问题,我们在此提供文章的摘要供您初步了解。如果您想要查阅更为详尽的内容,访问作者的公众号页面获取完整文章。


Large Language Models for Data Annotation: A Survey Summary
Introduction
Data annotation is a critical yet challenging step in machine learning and natural language processing, involving classification, addition of contextual tags, confidence scores, alignment or preference labels, entity relationships, semantic roles, and time series tagging. This process presents significant challenges due to the complexity, subjectivity, and diversity of data, requiring domain expertise and resource-intensive manual annotation. Advanced Large Language Models (LLMs) such as GPT-4 offer promising opportunities to innovate and automate data annotation tasks.
Contributions of the Survey
The survey focuses on the unique application of LLMs in data annotation, contributing insights into:
- LLM-based data annotation techniques and methodologies
- Evaluation of LLM-generated annotations
- Learning strategies utilizing LLM-generated annotations
- Challenges and ethical considerations in using LLMs for annotation
LLM's Role in Data Annotation
LLMs play a crucial role in improving the effectiveness and precision of data annotation processes, automating tasks, ensuring consistency across large datasets, and adapting to specific domains through fine-tuning or prompting, setting a new standard in the NLP field.
Methodologies and Learning Strategies
The survey delves into the nuances of using LLMs for data annotation, exploring methodologies, learning strategies, and associated challenges in this transformative approach. It aims to reveal the motivations behind using LLMs as catalysts for redefining the data annotation landscape in the fields of machine learning and natural language processing.
LLM's Potential in Diverse Scenarios
The research considers various settings such as fully supervised, unsupervised, and semi-supervised learning, addressing how LLMs can serve as annotators and the learning strategies based on their annotations. The scenarios share the annotation process by LLMs and the learning strategy based on these annotations.
Techniques and Adjustments with LLMs
The survey formalizes common techniques used in interactions with LLMs, including input-output prompts, context learning, Chain-of-Thought prompting, instructional adjustments, and alignment adjustments, which are essential for generating annotations and aligning LLM behaviors with human preferences.
Automated and Human-Centric Feedback
Aligning LLMs with human-centric attributes involves strategies that include human feedback and automated feedback mechanisms, leveraging other LLMs or the same LLM to evaluate outputs. This includes the use of reinforcement learning strategies and the evaluation of model-generated annotations.
Evaluation of LLM-Generated Annotations
Effective evaluation of LLM-generated annotations is crucial, with the survey addressing both general and task-specific assessment methodologies, as well as data selection through active learning.
Learning from LLM-Generated Annotations
The survey examines methodologies for learning from LLM-generated annotations, exploring direct use of annotations for domain reasoning, knowledge distillation to bridge LLMs with task-specific models, and fine-tuning and prompting techniques utilizing LLM annotations.
Challenges and Social Impact
LLM data annotation faces challenges including technical barriers, accuracy issues, and the social impact of labor displacement and bias propagation. Addressing these challenges is crucial for advancing LLM annotation applications.
Conclusion
This survey provides a systematic examination of methods, applications, and barriers related to the use of LLMs, including innovative strategies like prompting engineering and domain-specific adjustments. It evaluates the impact of LLM-generated annotations on training machine learning models and addresses technical and ethical issues.
Limitations and Ethical Statement
The survey acknowledges limitations like sampling bias and hallucinations in LLMs, dependence on high-quality data, complexity of adjustment and prompting engineering, and challenges in generalization and overfitting. It also commits to ethical principles such as fairness, transparency, privacy, human oversight, and social impact considerations.
想要了解更多内容?


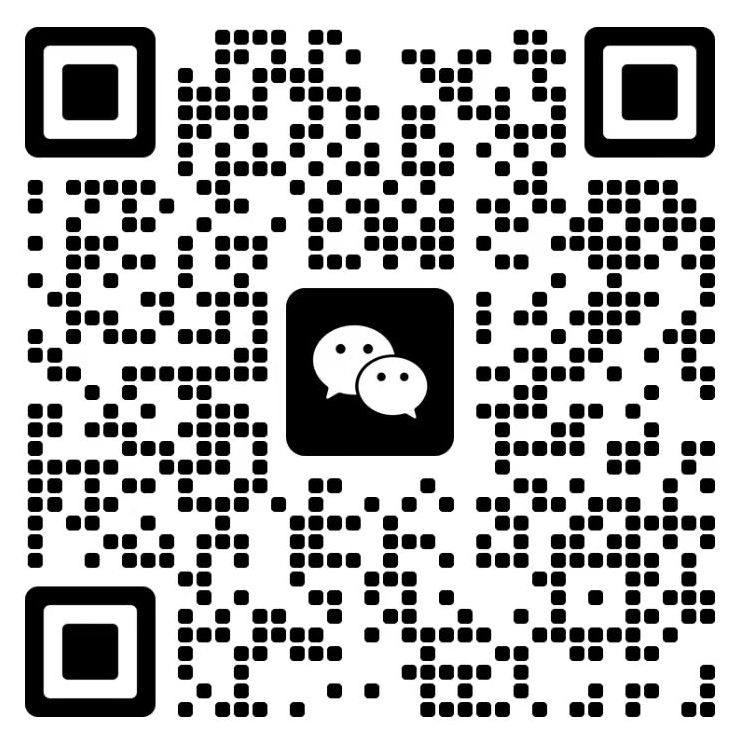
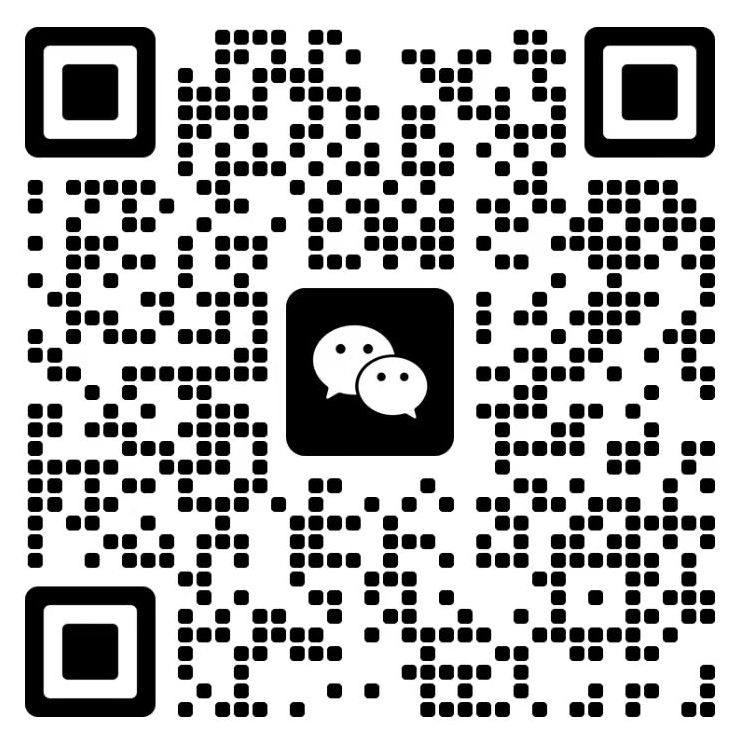