时隔5年,MobileNetV4重磅发布!

我们非常重视原创文章,为尊重知识产权并避免潜在的版权问题,我们在此提供文章的摘要供您初步了解。如果您想要查阅更为详尽的内容,访问作者的公众号页面获取完整文章。

MobileNet V4: A Leap Forward in Mobile Computer Vision
Five years after the release of MobileNet V3, Google has introduced MobileNet V4 (MNv4) to continue the series' commitment to balancing accuracy and efficiency in neural networks for mobile devices. The goal of MNv4 is to enhance users' experience on mobile devices by providing fast, real-time, and interactive capabilities.
The core advancements in MNv4 lie in the Universal Inverted Bottleneck (UIB) and the Mobile MQA modules, combined with an optimized Neural Architecture Search (NAS) recipe. UIB simplifies the architecture by integrating two optional depthwise convolutions (DW) within the inverted bottleneck block, enriching the architecture through NAS optimization. This integration unifies several important modules, including the original inverted bottleneck, ConvNext, and the Feedforward Networks (FFN) from Vision Transformers (ViT), also introducing a new variant called ExtraDW. The flexible construction of inverted bottleneck structures through NAS eliminates the need for manual scaling rules and achieves parameter sharing across different instantiations, leading to highly efficient NAS.
Complementing UIB, Mobile MQA is an innovative attention block designed specifically for accelerators, achieving up to 39% inference acceleration. An optimized NAS recipe further enhances the efficacy of MNv4 searches. The combination of UIB, Mobile MQA, and refined NAS has led to the creation of novel MNv4 models that exhibit exceptional performance across mobile CPUs, DSPs, GPUs, and dedicated accelerators such as the Apple Neural Engine and Google Pixel EdgeTPU.
A landmark achievement of MNv4 is its performance on the Pixel 8 EdgeTPU, where it attained an 87% ImageNet-1K accuracy with only 3.8 milliseconds of latency, marking significant progress in mobile computer vision capabilities. This development is an encouraging sign for those interested in the field, with the paper and code already accessible online.
For more information and access to the resources, the PDF of the paper can be found at arXiv and the code is available on GitHub.
想要了解更多内容?

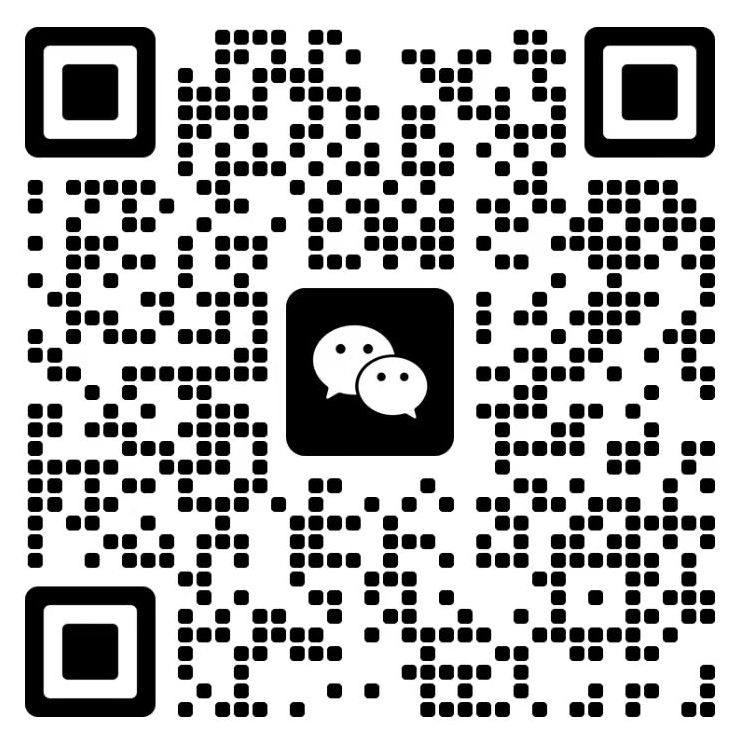
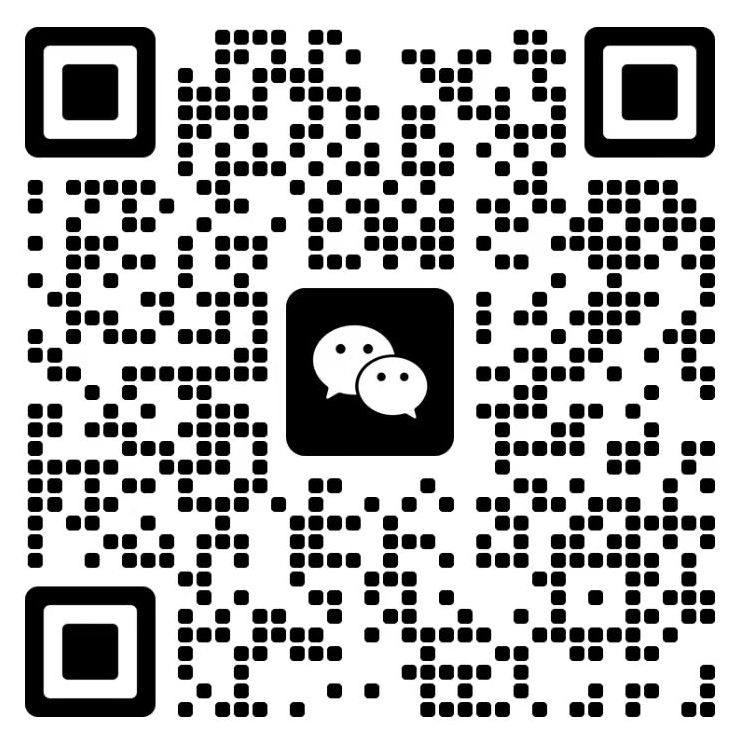