一文读懂数据平台建设的演进历程

我们非常重视原创文章,为尊重知识产权并避免潜在的版权问题,我们在此提供文章的摘要供您初步了解。如果您想要查阅更为详尽的内容,访问作者的公众号页面获取完整文章。


Summary of Data Platform Development Stages and Indicators
The article discusses the evolution of data platforms through various maturity levels, highlighting key indicators and considerations at each stage.
Level 0
At Level 0, data reporting is performed by directly querying transactional systems using tools like Excel or Power BI. This approach is quick but prone to issues related to data structure, source performance, and latency.
Level 1
Level 1 addresses performance issues with analytical queries in transaction systems by extracting data to separate databases or files, often during periods of low activity in the systems to mitigate disruption.
Level 2
Organizations at Level 2 combat inconsistent data and quality issues by establishing a data warehouse, which serves as a unified source for data analysis, ensuring consistent meaning across metrics.
Level 3
At Level 3, traditional data warehouses may be insufficient for handling large-scale, real-time, and diverse data sources. Introduction of data lakes addresses these challenges by allowing cost-effective and efficient storage of large volumes of data, including unstructured data, facilitating rapid integration and analysis of new data sources.
Level 4
Level 4 utilizes advanced technologies like data lakes or lake-house architectures to store large datasets in modern formats like Delta Lake, Iceberg, Apache Hudi, or Parquet. Real-time data analysis and machine learning models become pivotal in decision-making processes, enhancing predictive capabilities.
Various machine learning models are employed for tasks such as classification, regression, clustering, and time series prediction. The deployment process involves defining use cases, data acquisition, preparation, model training, and deployment, with tools available in both open-source libraries and cloud services.
Level 5
Although Level 5 is not yet fully realized, the continuous innovation in AI, especially generative AI, is expected to further influence the development of data platforms, enabling effortless interaction with AI for insights and decision-making. The intersection of AI capabilities and cost-effectiveness will define the boundaries of this transformative journey.
Data Mesh and Data Fabric
The article also touches upon emerging architectural trends like Data Mesh and Data Fabric. Data Mesh offers a decentralized model suitable for large organizations with multiple data teams, while Data Fabric centralizes data-related activities, potentially introducing bottlenecks in rapidly growing organizations.
Conclusion
The development of data platforms involves a combination of architecture methods, data governance, scalability, real-time and big data processing, and advanced analytics. Integrating machine learning and AI positions organizations at the forefront, catering to complex business needs and surpassing competitors. The extent of possibilities closely relates to an organization's size and development, with data literacy culture being essential internally.
想要了解更多内容?


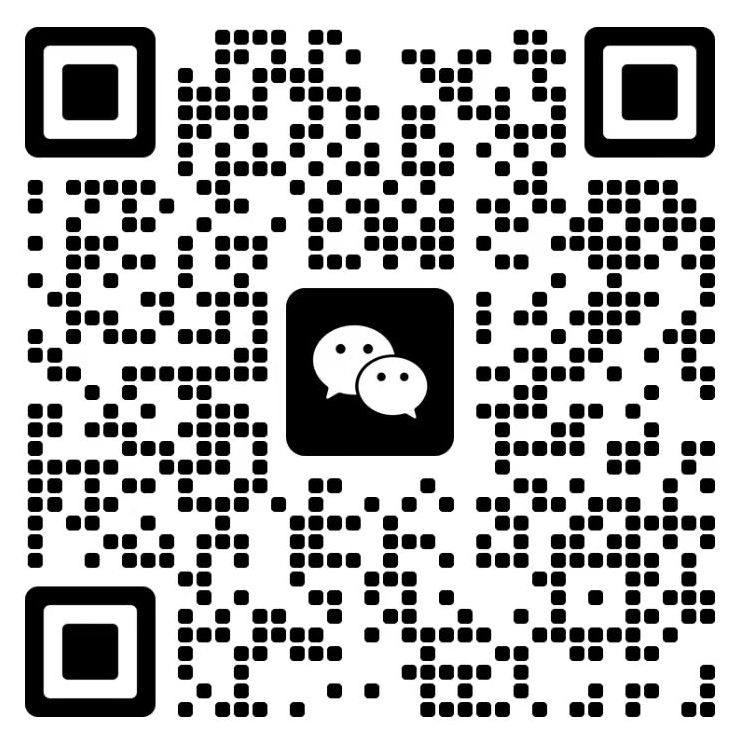
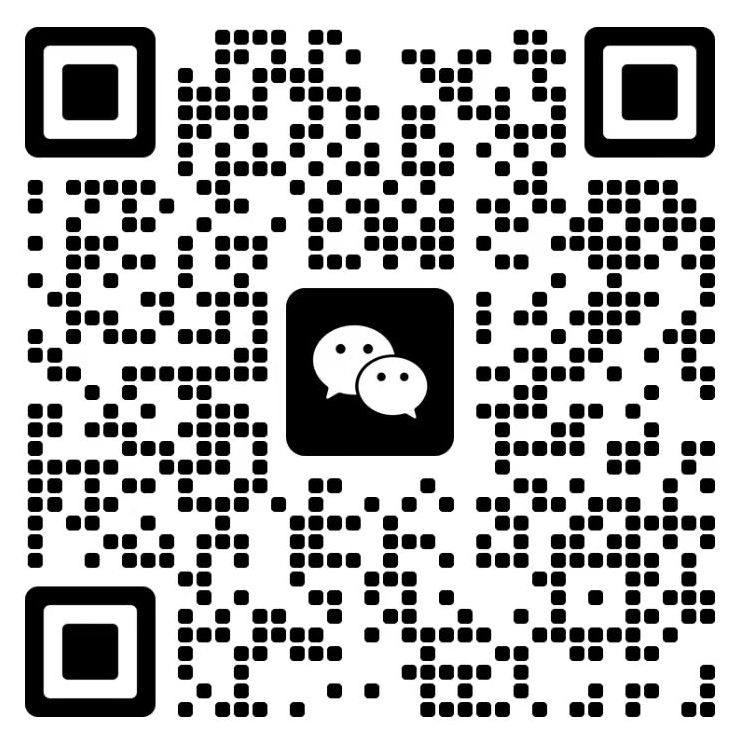